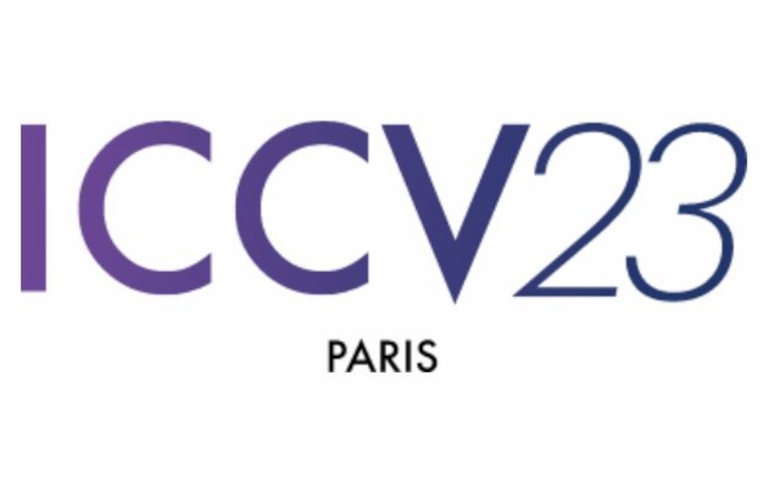
Paper presented at ICCV 2023 in Paris
9 Oktober 2023
Visual geo-localisation, i.e. finding a GPS coordinate without GPS, is a demanding problem in the computer vision community. To solve this problem, Siamese vision encoders are mostly used to assign the ground views without location to an aerial image with location. Since these Siamese vision encoders learn an assignment they benefit from so-called hard negatives, i.e. samples that are particularly difficult to distinguish from each other.
Imagine you're training a model to identify animals from images. If you show the model pictures of an elephant and a mouse, it will easily recognize that they are different – these are "easy negatives". However, if you show the model pictures of an African elephant and an Asian elephant, it will have a harder time distinguishing between them – these are "hard negatives". Training with "hard negatives" is more valuable as it challenges the model and makes it more precise.
In the article Sample4Geo: Hard Negative Sampling For Cross-View Geo-Localisation, Fabian Deuser, Konrad Habel and Norbert Oswald jointly investigated methods to select suitably difficult examples for training their algorithms. Their work introduced two sampling strategies for hard negatives. The first strategy harnesses geographically neighboring locations, while the second mines hard negative samples by leveraging the visual similarity between image embeddings. The developed methods outperform previous SOTA approaches on all relevant benchmarks. Due to its novelty, this work was accepted at the ICCV Conference 2023 in Paris and will be presented beginning of october.
Sample4Geo: Hard Negative Sampling For Cross-View Geo-Localisation
Fabian Deuser, Konrad Habel, Norbert Oswald