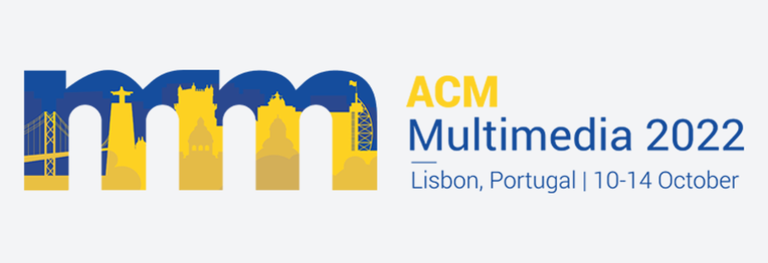
First place in the ACM 2022 MMSports Challenge
10 Oktober 2022
Computer vision meets team sports. Performance evaluation in the professional field is mainly done by experts evaluating the video material. Reviewing each material is time-consuming, especially if it is to be assessed at player level. For this purpose, player re-identification algorithms can help to find only relevant images and videos of a particular player.
In this paper, Konrad Habel, Fabian Deuser, and Norbert Oswald from the Institute of Distributed Intelligent Systems investigated contrastive approaches to better address this problem. Their research is based on OpenAI's groundbreaking paper (Radford et al., 2021) in multimodal zero-shot learning. In our work, we reformulated the pre-training task in CLIP into an image-to-image fine-tuning task based on the InfoNCE loss. With this approach, we obtained amazing results and won first place in the Player Reidentification Challenge of the MMSports Workshop at ACM Multimedia 2022.
CLIP-ReIdent: Contrastive Training for Player Re-Identification
Konrad Habel, Fabian Deuser, Norbert Oswald[arXiv], [ACM 2022], [MMSports Workshop], [ACM Paper]